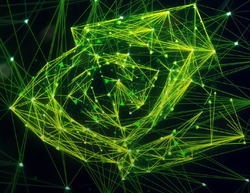
Nov. 13, 2018
By: Michael Feldman
Although NVIDIA isn’t announcing any new hardware at SC18, its GPUs continue to break new ground in the supercomputing space.
To begin with, the Department of Energy’s V100-accelerated Summit and Sierra supercomputers are now the top two systems in the world, at least when it comes to floating point prowess. Both systems improved on their TOP500 Linpack numbers from six months ago, widening Summit’s performance lead in its number one spot, and vaulting Sierra into the number two position. Each system added more than 20 petaflops to their Linpack total, bringing Summit to 143.5 petaflops, and Sierra to 94.6 petaflops. Those two systems alone represent nearly 17 percent of the total flops on the entire TOP500 list.
In fact, 702 of the 1,417 petaflops on the list are derived from accelerated systems, and the majority of those are based on NVIDIA’s P100 and V100 Tesla GPUs. One or the other of these GPUs are the basis for the most powerful systems in the US (DOE’s Summit), Europe (CSCS’s Piz Daint), and Japan (AIST’s AI Bridging Cloud Infrastructure), as well as the world’s most powerful commercial system (ENI’s HPC4).
NVIDIA GPU-accelerated supercomputers are also among the most energy-efficient. According to the Green500 list released on Monday, 8 of the 10 top ten and 22 of the top 25 most energy-efficient systems are equipped with NVIDIA silicon.
At least some of the company’s momentum can be attributed to the fact the V100 can accelerate both traditional 64-bit HPC simulations, as well as machine learning/deep learning (ML/DL) algorithms – the latter using the lower precision capabilities of the GPU’s Tensor Cores. While this might have seemed like an odd pairing a year or two ago, it turns out more and more HPC users are inserting ML/DL into their workflows. Once again, NVIDIA was ahead of the curve, much to the chagrin of its competition – AMD, Intel, et al.
This dual-acceleration advantage is reflected in the recent announcement of DGX-2 deployments at four DOE national labs. The DGX-2 is NVIDIA’s second-generation “AI supercomputer,” in this case, outfitted with 16 V100 GPUs connected via the high-performance NVSwitch networking fabric. Although the GPU-dense architecture is aimed specifically an ML/DL work, some of these labs are looking at using all this GPU horsepower for traditional HPC work as well.
For example, Brookhaven National Lab is using its new DGX-2 to do ML-based analysis on certain types of image recognition, but will also evaluate how they can exploit the system to accelerate some of their legacy high-energy physics and nuclear science codes.
Oak Ridge National Lab is employing its DGX-2 as an on-ramp to Summit, currently the largest GPU-accelerated supercomputer on the planet. This includes using the system to experiment with a variety of compute-intensive and data-intensive codes (including machine learning applications) that Oak Ridge users are interested in accelerating on Summit.
Pacific Northwest National Laboratory has a couple of specific applications in mind for its DGX-2 system, including atmospheric simulation codes, to model things like hurricane intensity, and whole-body millimeter wave scanning technology, to improve airport safety reduce false alarms.
Sandia National Laboratories is intending to use its DGX-2 as the foundation of its “Machine Learning as a Service.” The service is being developed as a user portal that encapsulates subject matter support for a range of science and engineering codes. The goal is to enable researchers with little expertise in ML/DL technology to use it to solve their domain-specific problems.
Ian Buck, NVIDIA’s GM and VP of Accelerated Computing, says we’re witnessing a new kind of HPC, where simulations, machine learning/deep learning, and other types of data analytics are forming a new paradigm for science and engineering. Some of this is being driven by the deluge of data streaming off scientific instruments and other edge devices, not to mention the data being generated by simulations themselves. The other facet to this is that Moore’s Law can no longer be counted on to provide the kind of performance increases that all these applications are now demanding.
Says Buck: “We’re entering a new era.”