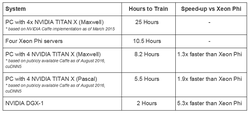
Aug. 16, 2016
By: Michael Feldman
In a blog post by NVIDIA’s Ian Buck, the CUDA creator admonished Intel for some of the GPU-bashing the chipmaker engaged in when it launched Knights Landing at the ISC 2016 conference. During the event, Intel rolled out a number of deep learning performance results that cast the NVIDIA hardware in a bad light against its latest Xeon Phi processor. But as Buck pointed out, Intel invariably made those comparisons against older GPU technology.
We here at TOP500 News also noted the apples-to-oranges performance comparisons Intel offered back in June, and predicted “we can look forward to a more level playing field in the coming months, which will pit NVIDIA’s Pascal GPUs against Intel’s Knights Landing chips.” Buck started down that path this week by offering some new numbers using its Pascal GPUs and some of the latest deep learning software.
Specifically, Buck refuted Intel’s assertions that Knights Landing is 2.3 times faster in training than GPUs, offers 38 percent better scaling than GPUs, and scales to 128 nodes, while GPUs do not. For the speed claims, he writes:
"Intel used Caffe AlexNet data that is 18 months old, comparing a system with four Maxwell GPUs to four Xeon Phi servers. With the more recent implementation of Caffe AlexNet, publicly available here, Intel would have discovered that the same system with four Maxwell GPUs delivers 30% faster training time than four Xeon Phi servers. In fact, a system with four Pascal-based NVIDIA TITAN X GPUs trains 90% faster and a single NVIDIA DGX-1 is over 5x faster than four Xeon Phi servers."
Source: NVIDIA
Now that last comparison is not exactly fair, given that the DGX-1 is equipped with eight Pascal P100 GPUs, not four. Nevertheless, the results point to a performance advantage for the NVIDIA parts, which is understandable given that the Maxwell and Pascal GPUs incorporate features optimized for deep learning. Also, since the Pascal Titan X chip delivers about 11 raw teraflops of single precision (FP32) performance versus about 6 teraflops for Knights Landing, it makes sense that the GPUs would come out on top for compute-intensive deep learning codes.
Regarding the better scaling claims made by Intel, Buck noted that the comparison was based on Oak Ridge Lab’s Titan supercomputer, which uses a four-year-old Kepler-era graphics processor, namely the Tesla K20X, as well as an older interconnect. Using a more recent cluster powered by Maxwell GPUs, Baidu was able to scale its speech training workloads almost linearly to 128 GPUs.
To Intel’s credit, it didn’t obscure the fact that it was comparing its Knights Landing with older technology. The company had meticulously footnoted all hardware and software configurations in its benchmark results. If anything, it was a sin of omission to ignore the fact the Pascal GPUs were the true counterparts to the Knights Landing processors. I suppose one could argue that the new NVIDIA processors didn’t exist two months ago, since technically they weren’t yet shipping. But in the scheme of things, both platforms are entering the market at essentially the same time.
Going forward, we’re likely to see a series of claims and counter-claims in the Knights Landing-Pascal GPU battles. And Intel is not without ammunition in this regard. The Xeon Phi includes a number of unique features, such as an integrated network fabric and standalone operation, which software should be able to exploit for all sorts of acceleration, AI or otherwise. And now that Intel is investing a third of a billion dollars in deep learning startup Nervana, the chipmaker may be able to incorporate some additional interesting IP into its Xeon Phi lineup down the road. As Buck points out, “few fields are moving faster right now than deep learning,” and that means no one has a lock on this market.