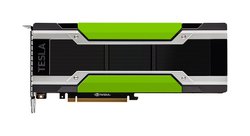
June 20, 2016
By: Michael Feldman
NVIDIA used the opening of the ISC High Performance conference (ISC) on Monday to launch its first Pascal GPU targeted to high performance computing. The announcement follows on the heels of the introduction of the Pascal P100 at the GPU Technology Conference in April, a device which was aimed at the deep learning market. The new HPC GPU, however, differs from its deep learning sibling in some surprising ways.
Confusingly, both products will carry the Tesla P100 name. The original product for deep learning will support NVIDIA’s NVLink inter-processor interconnect as well as PCIe, while the HPC version will support PCIe only. The logic behind this is that deep learning codes exhibit strong scaling, that is, the performance of these applications scale quite well with the number of processors used, in this case, GPUs. Since the principal advantage of NVLink is that it speeds memory accesses between GPUs in a server, the application space that stand to benefit the most from this is deep learning. NVIDIA realizes there are traditional HPC applications that can also make good use of servers equipped with many GPUs, they just represent a minority of that market.
A subtler aspect to this is that NVLink can also speed memory accesses between the CPU and the GPU – NVLink is about 5 -12 times faster than a PCIe 3.0 connection -- but only IBM, with its Power8+ chip, currently has a processor that offers such support. Most HPC servers today are based on x86 CPUs, and more that 90 percent of those are using Intel Xeon parts. Given that Intel is at war with NVIDIA, it is highly unlikely that a Xeon processor would ever support NVLink. But since PCIe is a standard interconnect, it acts as a sort of demilitarized zone here. As such, NVIDIA GPUs and Intel CPUs can pass data back and forth to each other over a PCIe bus unencumbered by business rivalry.
At some point in the not-too-distant future, we should see NVLink-capable GPUs for HPC, either in future Pascal GPU products, or in the next-generation Volta GPUs. Those Volta processors for HPC will certainly include NVLink since that particular feature has been promised to the DOE for the Summit and Sierra supercomputers, two future 100-petaflop-plus systems that the agency procured through the CORAL program.
Besides the discrepancy in NVLink support, the other big difference between the two P100 products is in the performance realm. The one targeted for deep learning is actually a faster processor since it’s clocked at a higher frequency (1.48GHz), providing a peak double precision performance of 5.3 teraflops. The HPC variant has a lower clock rate (1.3GHz) and tops out at 4.7 teraflops, making it about 11 percent slower. Single precision and half precision performance are also proportionally slower relative to the performance available in the deep learning GPU. The rationale was to get the HPC part down to 250 watts so that it would be better suited to the thermals of standard HPC servers, and that was accomplished with the marginal reduction in GPU clock speed.
The deep learning P100, on the other hand, runs at a toasty 300 watts, which apparently is just fine for the more specialized server gear used for these applications. Although these devices are mainly headed for deployment at hyperscale companies, they are not being installed at the volume we normally associate with the Googles and Facebooks of the world. Rather they are part of relatively modest-size clusters that are devoted to training the deep learning models, the most computationally intensive part of the process. In many cases, these servers are equipped with multiple GPUs and require specialized cooling infrastructure.
Most everything else in the new HPC P100 matches its deep learning twin, right down to its 16nm FinFET transistors. Bandwidth to the local memory is the same for both products: 720 GB/second for the 16 GB configuration. The high transfer rate is courtesy of the new second-generation High Bandwidth Memory (HBM2), a stacked semiconductor technology that offers much higher performance and densities than conventional graphics memory. The HPC part also offers a 12 GB configuration, which maxes out at 540 GB/second.
Although some supercomputing users may envy the faster computational speed of the deep learning GPU, an 11 percent performance hit on the HPC parts is probably a good tradeoff for the amount of power and cooling saved. It’s also worth pointing out that the HPC P100 is quite a bit speedier than its predecessor, the K80, which was based on the now-ancient Kepler architecture. The K80 peaks at 2.9 teraflops and 480 GB/second of bandwidth. And since it took two 28nm GPU chips to power that device, it drew a full 300 watts at top speed.
The new P100 also inherits the other goodies of the Pascal architecture. One of the most significant is its page migration engine, which is a hardware assist that allows applications to treat GPU and CPU memory as a unified address space. Pascal also comes with compute preemption, a facility that one normally associates with CPUs; it provides a more granular form of task switching than that of older GPU architectures.
The Bottom Line
Despite NVIDIA’s recent enthusiasm for deep learning, the HPC P100 shows that the company still takes the traditional HPC market very seriously. And with Intel’s Knights Landing Xeon Phi now shipping, NVIDIA is motivated to get its most capable silicon to market. From a raw performance perspective, the P100 stacks up well against the new Xeon Phi processors, outrunning them in both peak FLOPS and memory bandwidth. It also supports about 400 major HPC applications that have been ported to NVIDIA’s GPU processors over the past ten years. That, of course, has to be balanced by the Xeon Phi’s ability to provide x86 compatibility, and do so as a standalone processor.
The P100 GPUs for HPC are sampling today and will be generally available in the fourth quarter of 2016. One of the first systems to demonstrate the new silicon will be Piz Daint, the 7.8 petaflops supercomputer at the Swiss National Supercomputing Center (CSCS) in Lugano, Switzerland, which is expected to install 4,500 of the new GPUs as soon as they become available. Cray, IBM, Dell, and HPE will be shipping systems with the new GPUs before the end of the year. One would expect other HPC OEMs and system providers to follow suit further down the road.