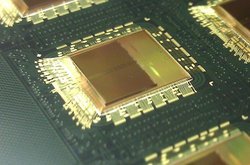
June 29, 2016
By: Michael Feldman
One of the most popular sessions at last week’s ISC High Performance conference was titled "Scaling Beyond the End of Moore’s Law," which was a series of three talks that delved into some of the technology options that could reanimate computing after CMOS hits the wall sometime in the next decade. The subject’s popularity is unsurprising, given that the supercomputing digerati that attend this event are probably more obsessed with Moore’s two-year cadence of transistor shrinkage than any other group of people on the planet.
But one couldn’t help coming away from the session thinking that, while there are a number of very compelling computing technologies on the horizon, none seem to have the capacity to replace Moore’s Law. That has more to do with the special nature of Moore’s Law, rather any shortcomings of the potential of the alternative technologies discussed in the session. The three presented technologies include quantum computing, presented by Damian Steiger from ETH – Zurich; neuromorphic computing, presented by Karlheinz Meier from the University of Heidelberg; and what might be described as “heroic CMOS,” presented by Intel’s Josh Fryman.
Fryman’s talk began by describing the purpose behind Moore’s Law, which he characterized as a “business statement,” as opposed to any sort of technology projection. From Intel’s point of view, this has certainly been true, inasmuch as the company’s financial success has been predicated on consistently providing its customers more transistors for the same price every 24 months or so. Left unsaid was what becomes of your business when your business statement fails.
Next Fryman took the session attendees on a brief historical walk through the various technologies used to advance electronics over the years, starting with electro-mechanical in the 19th century, and proceeding through electronic-VT, bipolar, NMOS, and finally CMOS. The implication here is that another technology is waiting to be discovered that will grab the baton from CMOS once the physics of transistor shrinking becomes untenable. But Fryman didn’t offer any such candidates, despite Intel’s own forays into research areas like carbon nanotubes and spintronics.
His focus, rather, was on ways to lengthen the useful lifetime of CMOS. He talked about areas like Near Threshold Voltage (NTV), a technology Intel was quite excited about a few years ago, since it provides significantly better execution throughput per watt by reducing supply voltage. It also enables denser transistor geometries, since energy leakage is minimized. Experimental chips bore this out, but the technology didn’t pan out, presumably because the single-threaded performance levels achieved were deemed inadequate for most computing applications.
Another technology known as fine-grain power management, where certain regions of the chip are put to sleep when they are not being used, is actually being implemented by most chipmakers and, according to Freyman, can deliver 60 percent better efficiency. Other approaches, which include additional fiddling with the depth of the instruction pipeline or increasing speculative execution, have already been tried, but they involve pretty significant tradeoffs between performance, die area and energy efficiency.
One gets the impression that the obvious CMOS refinements have already been largely exploited, and what is needed is a what Freyman proposed at the start of his talk: a post-CMOS technology that will take the industry into a post-Moore realm. While he admits that there is no such technology on the horizon and that it would take 10 to 15 years of R&D to commercialize once it once it was discovered, Freyman maintains optimism that a solution will eventually be found.
Moving on to neuromorphic computing portion of the session, Karlheinz Meier described how this technology is being used to mimic certain brain functionality, such as pattern recognition and other types of complex data correlations and inferences. Essentially it uses our knowledge about how neurons and synapse operate in the biological realm and applies that to computers, both in hardware and software.
Meier described SpiNNacker, a project out of the University of Manchester, which is developing a million-core ARM processor-based system that aims to simulate a billion neurons. A 48-chip board has been built and will serve as the building block for larger systems. To reach a neural network of the size envisioned, it will take more than 10,000 such boards.
Also described was a second project, known as BrainScaleS, which Meier heads at the University of Heidelberg. The hardware platform uses customized ASICs plus FPGAs to simulate a neural network. The underlying hardware for SpiNNaker and BrainScaleS is based on current semiconductor technology, but Meier believes the neural network model is well-suited for “deep-submicron, non-CMOS devices”, although this is not the focus of his efforts.
After 10 years, Meier believes that the project work has reached a certain level of maturity, at least enough to tackle what he calls “non-expert use cases.” he admits that the technology is not applicable to general-purpose computing, but will have broad applicability in the emerging application set that falls under the category of machine learning. Other application areas await discovery.
Switching to the quantum realm, Damian Steiger began by talking about some of the major challenges in developing a general-purpose quantum computer, which mainly revolve around building these slippery little things called qubits. The main issues here are stabilizing the qubits long enough to be of practical use and assembling enough of them in a system so as to have a reasonably sized machine.
He described different experimental versions of qubits (along with their strengths and weaknesses), including, ion traps (stable, but slow and hard to scale), superconducting qubits (scalable and fast, but short lifetimes), and something called topological qubits, which are intrinsically protected from noise as a consequence of their configuration. There’s also promising new research in the area of Majorana particles, which some feel can be utilized as the basis for these types of quantum devices.
Like neuromorphic computing, quantum computing is not a replacement for general-purpose computing, but has the property of being able to solve certain types of problems that aren’t amenable to solutions on digital hardware in any reasonable timeframe. That includes esoteric applications like cracking 1024-bit RSA encryption, but Steiger also envisions quantum computing can be applied to more down-to-earth problems in materials science, chemistry, nanoscience, and machine learning.
For example, he sees it being used for designing room-temperature semiconductors, developing a catalyst for carbon sequestration, and finding better mechanism for nitrogen fixation. Doing these with classical algorithms is possible, but as the models for these simulations get larger and more complex, computation time grows exponentially. Quantum computers can solve these types of applications more elegantly.
At this point, it is worth pointing out that neither neuromorphic computing nor quantum computing replaces CMOS technology, or for that matter, digital computing in general. They are merely different paradigms for data processing. More to the point, they do not represent technology paths, as Moore’s Law does, just technologies. Even the aforementioned carbon nanotubes and spintronics would fall into that category.
Until one of these technologies is developed to a reasonable degree, it is unlikely that a well-defined roadmap for it will magically spring into place. In fact, some technologies will end up being one-hit wonders, where any advancement comes through painstaking refinements, with the occasional breakthrough, rather than through any well-defined scaling model. The general category of software is a rather visible example of a non-scalable computing technology. So the best we can do today is probably what Freyman recommended, namely to keep searching for that technology on the other side CMOS. Until then, we can enjoy the ride on Moore’s Law… while it lasts.