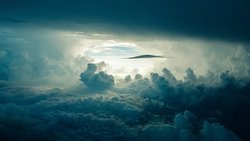
Dec. 12, 2018
By: Michael Feldman
A group of researchers are using deep learning to improve the speed and accuracy of supercomputer-powered climate simulations.
The work, which is being performed by researchers at the University of California, Irvine, the Ludwig Maximilian University of Munich and Columbia University, is focused on training neural networks to more accurately predict the how clouds are driving the Earth’s weather patterns, and by extrapolation, the longer-term climate effects. The resulting model, known as the “Cloud Brain,” was integrated into a traditional climate simulation with the hopes of improving its fidelity and performance.
The problem with physics-based climate models is that their resolutions are too coarse to accurately capture the behavior of the atmosphere. That’s because even with the multi-petaflop supercomputers of today, it’s much too time-consuming to simulate all the atmospheric components with high granularity. That is particularly true in the case of clouds, which are inherently small-scale phenomenon.
According to an article published this week by Yale Environment 360, the limitations of the traditional approach has led to a situation where the results of the various climate models used by Intergovernmental Panel on Climate Change (IPCC) are significantly different from one another. For example, if the models assume a doubling of the carbon dioxide in the atmosphere, one model will predict a 1.5-degree warmup, while another will forecast a 4.5-degree increase. “It’s super annoying,” says Michael Pritchard, one of the Cloud Brain developers at the University of California, Irvine (UCI) who is quoted extensively in the article.
The neural network developed by Pritchard and his colleagues was trained to predict the effects of clouds using thousands of high-resolution models, which could then be applied to the larger-scale simulations. When the project was announced by UCI back in September, Pritchard noted that it took just three simulated months of model output to train the original Cloud Brain network.
Stephan Rasp, an LMU doctoral student in meteorology who began collaborating with Pritchard on this project as a visiting scholar at UCI, explained the significance of the work thusly: “The neural network learned to approximately represent the fundamental physical constraints on the way clouds move heat and vapor around without being explicitly told to do so, and the work was done with a fraction of the processing power and time needed by the original cloud-modeling approach.”
In the ensuing research paper authored by Rasp, Pritchard, and Columbia University professor Pierre Gentine, they noted that the trained neural network was able to replace the traditional (coarse) cloud parameterizations, while successfully interacting with other elements of the simulation. The results were promising.
“The prognostic multiyear simulations are stable and closely reproduce not only the mean climate of the cloud-resolving simulation but also key aspects of variability, including precipitation extremes and the equatorial wave spectrum,” wrote the authors.
And by virtue of the inherent efficiency of trained neural networks, it was able to speed up these parameterizations by a factor of 20, saving precious supercomputer time. As the researchers noted, a key advantage to the use of deep learning is that even with a more refined neural network based on higher resolution data, the runtime savings are maintained.
The Cloud Brain is still a research project and, as documented in the Yale Environment 360 article, there are a number of hurdles to overcome. One of the more stubborn of these – and one that permeates deep learning more generally – is that a neural network operates as a black box, with no clear indication of how it generates its predictions. For forecasting a future climate, where there are no definitive results to compare against, this approach is difficult to trust.
Nevertheless, the researchers intend to extend their work to new models, while also trying to overcome some of its limitations. “Our study shows a clear potential for data-driven climate and weather models,” said Pritchard. “We’ve seen computer vision and natural language processing beginning to transform other fields of science, such as physics, biology and chemistry. It makes sense to apply some of these new principles to climate science, which, after all, is heavily centered on large data sets, especially these days as new types of global models are beginning to resolve actual clouds and turbulence.”