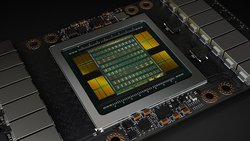
June 26, 2018
By: Michael Feldman
For the first time in history, most of the flops added to the TOP500 list came from GPUs instead of CPUs. Is this the shape of things to come?
Tesla V100 GPU. Source: NVIDIA
In the latest TOP500 rankings announced this week, 56 percent of the additional flops were a result of NVIDIA Tesla GPUs running in new supercomputers – that according to the Nvidians, who enjoy keeping track of such things. In this case, most of those additional flops came from three top systems new to the list: Summit, Sierra, and the AI Bridging Cloud Infrastructure (ABCI).
Summit, the new TOP500 champ, pushed the previous number one system, the 93-petaflop Sunway TaihuLight, into second place with a Linpack score of 122.3 petaflops. Summit is powered by IBM servers, each one equipped with two Power9 CPUs and six V100 GPUs. According to NVIDIA, 95 percent of the Summit’s peak performance (187.7 petaflops) is derived from the system’s 27,686 GPUs.
NVIDIA did a similar calculation for the less powerful, and somewhat less GPU-intense Sierra, which now ranks as the third fastest supercomputer in the world at 71.6 Linpack petaflops. And, although very similar to Summit, it has four V100 GPUs in each dual-socked Power9 node, rather than six. However, the 17,280 GPUs in Sierra still represent the lion’s share of that system’s flops.
Likewise for the new ABCI machine in Japan, which is now that country’s speediest supercomputer and is ranked fifth in the world. Each of its servers pairs two Intel Xeon Gold CPUs with four V100 GPUs. Its 4,352 V100s deliver the vast majority of the system’s 19.9 Linpack petaflops.
As dramatic as that 56 percent number is for new TOP500 flops, the reality is probably even more impressive. According to Ian Buck, vice president of NVIDIA's Accelerated Computing business unit, more than half the Tesla GPUs they sell into the HPC/AI/data analytics space are bought by customers who never submit their systems for TOP500 consideration. Although many of these GPU-accelerated machines would qualify for a spot on the list, these particular customers either don’t care about all the TOP500 fanfare or would rather not advertise their hardware-buying habits to their competitors.
It’s also worth mentioning that the Tensor Cores in the V100 GPUs, with their specialized 16-bit matrix math capability, endow these three new systems with more deep learning potential than any previous supercomputer. Summit alone boasts over three peak exaflops of deep learning performance. Sierra’s performance in this regard is more in the neighborhood of two peak exaflops, while the ABCI number is around half an exaflop. Taken together, these three supercomputers represent more deep learning capability than the other 497 systems on the TOP500 list combined, at least from the perspective of theoretical performance.
The addition of AI/machine learning/deep learning into the HPC application space is a relatively new phenomenon, but the V100 appears to be acting as a catalyst. “This year’s TOP500 list represents a clear shift towards systems that support both HPC and AI computing,” noted TOP500 author Jack Dongarra, Professor at University of Tennessee and Oak Ridge National Lab.
While company’s like Intel, Google, Fujitsu, Wave Computing, Graphcore, and others are developing specialized deep learning accelerators for the datacenter, NVIDIA is sticking with an integrated AI-HPC design for its Tesla GPU line. And this certainly seems to be paying off, given the growing trend of using artificial intelligence to accelerate traditional HPC applications. Although the percentage of users integrating HPC and AI is still relatively small, this mixed-workflow model is slowly being extended to nearly every science and engineering domain, from weather forecasting and financial analytics, to genomics and oil & gas exploration.
Buck admits this interplay between traditional HPC modeling and machine learning is still in the earliest stages, but maintains “it’s only going to get more intertwined.” He says even though some customers will use only a subset of the Tesla GPU's features, the benefits of supporting 64-bit HPC, machine learning, and visualization on the same chip far outweighs any advantages that could be realized by single-purpose accelerators.
And, thanks in large part to these deep-learning-enhanced V100 GPUs, mixed-workload machines are now popping up on a fairly regular basis. For example, although Summit was originally going to be just another humongous supercomputer, it is now being groomed as a platform for cutting-edge AI as well. By contrast, the ABCI system was conceived from the beginning as an AI-capable supercomputer that would serve users running both traditional simulations and analytics, as well as deep learning workloads. Earlier this month, the MareNostrum supercomputer added three racks of Power9/V100 nodes, paving the way for serious deep learning work to commence at the Barcelona Supercomputing Centre. And even the addition of just 12 V100 GPUs to the Nimbus cloud service at the Pawsey Supercomputing Centre was enough to claim that AI would now be fair game on the Aussie system.
As Buck implied, you don’t have to take advantage of the Tensor Cores to get your money’s worth from the V100. At seven double-precision teraflops, the V100 is a very capable accelerator for conventional supercomputing. And according to NVIDIA, there are 554 codes ported to these graphics chips, including all of the top 15 HPC applications.
But as V100-powered systems make their way into research labs, universities, and commercial datacenters, more scientists and engineers will be tempted to inject AI into their 64-bit applications. And whether this turns out to be a case of the tail wagging the dog or the other way around, in the end, it doesn’t really matter. The HPC application landscape is going to be forever changed.
….