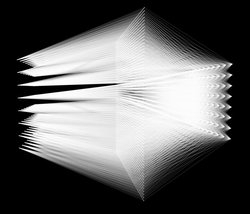
Feb. 21, 2018
By: Michael Feldman
Silicon Valley startup Fathom Computing has announced that it is building an optical computer that can train large-scale neural networks much more efficiently than GPUs.
If that introduction invokes some déjà vu, that’s because we recently wrote about another optical computing startup, called Lightmatter, which is using its custom-built silicon photonics-powered processor to go after this same market. Besides Lightmatter and Fathom Computing, there are at least a couple of other companies taking aim at the AI/machine learning with optical computing, including LightOn, a Paris-based startup that recently began testing its technology in a datacenter, and Lightelligence, an MIT spinout backed by Baidu Ventures.
In the case of Fathom Computing, the company appears to be building an entire optical computing system for accelerating deep learning applications. The company recently demonstrated a prototype system that trained several deep neural networks, including long- short-term memory recurrent neural networks and feedforward networks. “This is the first demonstration of programmable multi-layer neural network training on an optical compute,” says the website. “This optical architecture has remarkable potential for scaling to very large neural networks.”
Like other optical computing proponents, the company points out that light has an inherent advantage in performance and energy efficiency over electronic transistors. Fathom claims that its hardware can “significantly outperform state-of-the-art GPUs, adding that “a single Light Processing Unit (LPU) has the potential to replace the deep learning work of an entire supercomputer.”
The first Fathom systems are slated to debut in a couple of years and are intended to be deployed in the cloud so that they can be easily accessible to AI researchers. Fathom’s longer-term goal is to build a platform that can “train artificial neural networks with the complexity and graph scale of the human brain.”
Image: Visualization of optical connectivity in Fathom Computing system