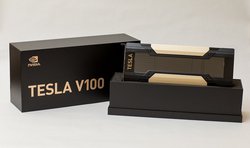
Sept. 29, 2017
By: Michael Feldman
The world’s largest server OEMs announced they will be soon be shipping systems equipped with NVIDIA’s latest Volta-generation V100 GPU accelerator. Included in this group are Hewlett Packard Enterprise (HPE), Dell EMC, IBM, Supermicro, Lenovo, Huawei, and Inspur, all of which took the opportunity to reveal their Volta-powered servers at this week’s GPU Technology Conference in China.
Source: NVIDIA
The V100 has been shipping for a few months, but until now deployments were restricted to NVIDIA’s own DGX-1 appliance and DGX Station offerings, which have initially found their way into a handful of AI research organizations. The addition of these major OEMs will enable NVIDIA’s flagship GPU accelerator and deep learning wunderkind to find a much broader customer base, in particular, hyperscale cloud providers and supercomputing centers.
Starting with HPE, its new V100 servers will encompass both the Apollo and Proliant platforms. In the latter case, ProLiant DL380 will support up the three V100 GPUs in a fairly typical two-socket server. It seems to be aimed at applications that require a modest amount of GPU support -- database acceleration, and things of that sort.
For more intense acceleration, HPE is offering the Apollo 6500, which can be equipped with up to eight V100 GPUs and two Xeon CPUs in its 2U enclosure. The 6500 can be configured in a number of ways, according to what sort of GPU-CPU balance you’re going for. For applications that are very GPU-intensive, like deep learning, you can connect four V100s to a PCIe switch, and two of these switches into a single CPU. For something that needs more CPU presence, say HPC workloads, you can hook four GPUs to a CPU. The system supports peer-to-peer GPU communications via GPUDirect, with four V100s per InfiniBand adapter.
The Dell EMC will be offering V100 support in its PowerEdge R740, R740XD, and C4130 servers. The R740 and R740XD can connect up to three V100 GPUs via PCIe, while the C4130 will support up to four V100 GPUs, using either PCIe or NVIDIA’s NVLink interconnect. For the NVLink capability, the SXM2 form of the V100 will be employed, offering 300 GB/second of GPU-to-GPU bandwidth. Besides the higher interprocessor bandwidth, the NVLink-powered SXM2 is also somewhat more performant than the PCIe-only V100, at least on paper, offering 7.8 versus 7.0 double precision teraflops, and 125 versus 112 deep learning teraflops, respectively.
Dell EMC is targeting these V100-accelerating PowerEdge servers at both deep learning and traditional high performance computing customers. Notably, the company ran a series of HPC benchmarks on different configurations of the C4130 server and found that that the NVLink-equipped V100s generally performed better. That was especially apparent on molecular simulation kernels LAMMPS and AMBER, although on the more contrived benchmarks of High Performance Linpack (HPL), and the High Performance Conjugate Gradients (HPCG) suite, the PCIe V100 actually did slightly better than the NVLink-enabled GPUs. Overall though, the new V100 servers were between 24 to 80 percent faster than those using the older Pascal-generation P100 GPUs.
IBM is also planning to support the V100 in its upcoming Power9 server line. At this point there isn’t much information on the particulars, which is understandable, given that the company has not even launched the Power9 chips yet. At this point, IBM is just saying that the new servers will incorporate “multiple V100 GPUs.” Since we already know that the Power9-V100 servers in the DOE’s Summit supercomputer will have 2 CPUs and 6 GPUs per node, it’s a decent bet that it’s going to support that many, or perhaps more, in its commercial offerings. Currently, IBM’s S822LC server for HPC can house up to four of the P100 GPUs.
What differentiates IBM’s design is that the Power9 chip will support NVLink natively, enabling the same sort of ultra-fast interprocessor communication between the CPU and GPU that only the GPUs themselves enjoy in Intel Xeon-hosted systems. How much better performance this delivers remains to be seen, but for applications that do a lot of CPU-GPU crosstalk, this feature should give these servers a edge.
Supermicro gear supporting the V100 GPUs include the 4028GR-TXRT, 4028GR-TRT, and 4028GR-TR2 servers, as well as the 7048GR-TR workstation. The servers are principally targeted at the deep learning space, while the workstation is aimed at desktop HPC. Supermicro will also be selling an V100-accelerated 1028GQ-TRT server for advanced analytics.
Lenovo, Huawei, and Inspur will be offering different variations of NVIDIA’s HGX reference platform, mainly for hyperscale datacenters doing deep learning-based web services. NVIDIA popularized the HGX design with its own DGX-1 server, as well as Microsoft’s Project Olympus HGX-1 server and Facebook’s Big Basin system. The standard HGX architecture is made up of eight NVLink-capable GPUs (the SXM2 form factor) connected in a cube mesh using NVLink. In this case, the new HGX implementations with the V100 will include Lenovo’s HG690X and HG695X servers, Inspur’s 2U-8GPUAGX-2 server and Huawei’s G-series heterogenous servers.
NVIDIA also announced today that Alibaba, Baidu and Tencent are planning to deploy the new V100 GPUs into their cloud datacenters. Who will be providing the servers for these deployments is still up in the air, but the aforementioned Lenovo, Huawei, and Inspur are now possibilities, as are ODMs Foxconn, Inventec, Quanta and Wistron. For what it’s worth, the P100-powered HGX-1 that Microsoft is using is manufactured by Foxconn subsidiary Ingrasys, and Facebook’s P100-based Big Basin hardware is built by Quanta.
The bottom line is that the V100 will soon be widely available, and likely, widely deployed across most of the major cloud service providers. Its footprint in supercomputing centers is much less certain. Besides the DOE’s Summit and Sierra systems, no major installations have been announced. Of course, the V100s are still at the beginning of their production cycle. And given their 7-teraflop performance and the increasing interest in embedding deep learning into HPC workflows, we’re likely to see quite a few of these GPUs in supercomputers before it’s all over.