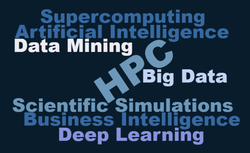
Oct. 18, 2017
By: Michael Feldman
Over the last several years, the realm of high performance computing has grown appreciably beyond its roots in scientific simulations. Today it encompasses a lot more data analytics, including emerging areas like artificial intelligence, as well as more established applications like business intelligence. But, if you’re an HPC market research company like Hyperion Research, tracking the high-end analytics space is no easy task.
As lead analyst for Hyperion’s High Performance Data Analytics (HPDA) segment, it’s Steve Conway’s job to make sense of these newer areas and how they fit into the broader HPC market. Recently, we got the opportunity to ask Conway about the challenge of defining and tracking the high-end analytics market and, at a higher level, how it is changing the nature of high performance computing.
TOP500 NEWS: As you track AI and machine learning, how do you keep them separate from seemingly related applications like business intelligence, predictive analytics and data mining – basically the remainder of your HPDA category? What’s your criteria?
Steve Conway: It's not simple, but we start with clear, definition-based rules for categorizing markets. We treat machine learning, deep learning and other AI categories as methodologies rather than markets, because they can be applied across multiple vertical market segments. As methodologies, they're analogous to computational fluid dynamics and finite element analysis rather than to verticals like life sciences or manufacturing.
Of the other things you mentioned, predictive analytics and data mining are also methodologies, but business intelligence is a market and we treat it that way. In the surveys we conduct about once a quarter, business intelligence has consistently been the fastest-growing big data analytics market for HPC.
TOP500 NEWS: How are you tracking AI/machine learning revenue? In other words, which application areas, customers, and hardware components fall under the HPC umbrella?
Conway: Bear with me here, but our hierarchy goes like this. HPC is the top category. Within the HPC market there are the 13 application-based vertical segments we've tracked for 25 years, such as life sciences, defense, energy, financial services and others. Within each of these, we split out the data-intensive component that we call high performance data analysis, or HPDA. That includes both data-intensive simulation and data-intensive analytics, because many HPC sites are now doing both, often on the same HPC system.
The newer additions to our HPDA category are repetitive use cases for commercial analytics, mostly involving commercial firms adopting HPC for the first time. The important ones here so far are fraud and anomaly detection, affinity marketing, business intelligence and precision medicine. Methods like machine learning or deep learning or graph analytics can be applied in just about any established or new HPC market segment.
Even though we treat machine and deep learning and AI in general as methodologies, we size and forecast these new opportunities separately because they've become important and there's a demand for us to do that.
TOP500 NEWS: Are you tracking other aspects of AI/machine learning beside server spending?
Conway: We track and forecast HPDA storage in the same depth as HPDA servers, right down to the split-outs by vertical. We've also done major studies for government agencies and private customers, including an extensive taxonomy of HPDA algorithms, tying their attributes to hardware-software requirements.
TOP500 NEWS: When you look at a system like Japan’s AI Bridging Cloud Infrastructure (ABCI), or essentially any system that is used for both traditional HPC work and machine learning, do you count those servers as AI spending or HPC?
Conway: We define HPC as the market for work performed on technical servers by scientists, engineers or data analysts. When aspects of machine learning are done on desktop computers, we don't count that as HPC. When the work moves to servers, we treat it as HPC. In our latest global survey of HPC users, 53 percent said they run simulation and analytics workloads on the same HPC system, so it doesn't make sense to treat simulation and analytics as separate markets.
TOP500 NEWS: On a related note, are you seeing HPC sites starting to run machine learning applications – either integrated into their traditional simulation workflows or as discrete standalone applications?
Conway: Yes. Both these practices are ramping up quickly, but they're not brand new. The HPC community was the birthplace of big data analytics, starting with the intelligence community in the 1960s and then joined by large investment banks in the 1980s. By the early 1990s, George Mason University Hospital in Washington, DC was routinely using a Cray supercomputer to help detect breast cancer after being trained to identify early indicators, called microcalcifications, on X-ray films with better-than-human ability. That was an early example of machine learning, and not the only one. So, machine learning has been a part of HPC for quite a while. It's just that it's catching fire now.
TOP500 NEWS: On another related note, given the non-double-precision math and non-linear-algebra math used in most of AI and machine learning, do you think the TOP500 list is still relevant to the HPC industry?
Conway: The TOP500list is very valuable as a census tracker for developments affecting the largest supercomputers over time, and for that purpose it will remain relevant. It was never intended to measure or predict the performance of supercomputers on a broad spectrum of real-world HPC applications, even though it's often misinterpreted as doing that. What we're seeing with exascale programs, especially in North America and Europe, is a shift toward targeting specific performance gains over today's biggest supercomputers on collections of real-world applications. NCSA helped set the stage for this shift by basing Blue Waters on carefully researched user requirements and not paying attention to Linpack results for the TOP500 ranking.
TOP500 NEWS: So, what kinds of revenue growth are you forecasting for HPDA servers and the subset of AI servers?
Conway: We forecast that worldwide revenue for HPDA servers will grow from $1.5 billion in 2016 to $4.0 billion in 2021. That's a 17 percent CAGR. Global revenue for the HPDA subset of AI servers, we predict, will expand at an even stronger 29.5 percent CAGR from $246 million in 2016 to $1.3 billion in 2021. Adding in storage, software and support service just about doubles each of these figures.
TOP500 NEWS: What’s the impact of that growth for the overall HPC market? In other words, without HPDA, what would the HPC market look like? And without AI, what would the HPDA sub-segment look like?
Conway: We've tracked HPDA as part of the HPC market since 2009 and we do the same now with HPC server-based AI, so the numbers we provide for the HPC market include HPDA and AI. Since we also track HPDA and AI numbers separately before baking them into the overall HPC market numbers, it's easy to see how much these fast-growing areas are contributing.
TOP500 NEWS: From a technology perspective, how do you think the emergence of AI/machine learning is impacting the direction of HPC hardware and software?
Conway: HPC systems have been hosting some of the world's most data-intensive workloads in simulation and analytics for decades, which is why more and more commercial businesses are acquiring HPC systems to run analytics workloads their enterprise servers can't handle alone. It's also why HPC has moved to the forefront of R&D in the AI-machine learning-deep learning domain, as well as for IoT. The recent powerful trend toward AI and other HPDA workloads is driving HPC system vendors to move away from compute-centric, "flop-sided" architectures toward more balanced designs with stronger memory and storage capabilities and faster data rates, and we see this trend continuing. Many of the new workloads need only single precision, or even half precision, and we expect to see more HPC systems purpose-built to excel on these workloads.