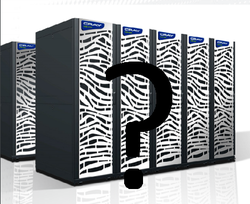
Oct. 10, 2016
By: Michael Feldman
As HPC vendors like IBM and HPE have added deep learning-optimized systems to their product portfolio, Cray has been more circumspect about its plans in this area. We talked with Cray CTO Steve Scott about how they view this new application area of high performance computing and what they may have in the pipeline to serve this burgeoning market.
If Cray, the quintessential HPC company, has a play here it’s because deep learning, at least the part that trains the neural networks, is a quintessential HPC application. It is both compute-intensive and data-intensive, demanding high performance processors, memory, networking, and I/O. And like the typical HPC application, it has insatiable demands for these resources. Larger deep learning problems requiring greater capability are relatively easy to devise.
There is certainly a market opportunity to provide deep learning clusters. Right now most of those customers are the hyperscale companies like Google, Amazon, Baidu, and Microsoft, but learning deep learning appears poised to move into the enterprise as well. In the second quarter of 2016, NVIDIA attributed nearly half its $151 million in datacenter sales to GPUs intended for deep learning. That a lot of GPUs, and they ended up in machines built by one server vendor or another.
At this point, Cray has no platform specifically optimized for deep learning, but according to CTO Scott, “we are actively exploring this area.” The company’s existing CS-Storm platform, a more-or-less conventional HPC cluster accelerated by up to eight GPUs per node, is the closest they have today. In fact, the CS-Storm brochure mentions machine learning as a suitable application target. But what Scott and company are developing sounds somewhat different – perhaps very different.
Scott said they are looking to build a system with node size, network to computing ratio, software stack, and density all optimized for this application set. The latter, especially GPU density, is certainly one of the most important aspects. Deep learning platforms available on the market today, like IBM’s S822LC for High Performance Computing and HPE’s Apollo 6500 system follow this general design of GPU-dense server nodes hooked together with InfiniBand or high performance Ethernet. IBM’s platform, for example, provides up to four GPUs per node, while HPE’s offering gives you up to eight GPUs per node. It’s almost certain Cray will follow suit with such GPU density, and perhaps even up the ante a bit.
It also likely that Cray will employ NVIDIA’s latest P100 GPUs in its deep learning machine. Scott recognizes that the half-precision support (FP16) in the Pascal platform will offer a significant advantage for such workloads. In addition, the NVLink capability in high-end P100 offers a significant performance advantage in GPU-to-GPU crosstalk within a node. It’s conceivable that Cray could opt for the non-NVLink (PCIe-only) version of the P100 to contain costs, but that seems like a remote possibility if the goal is to maximize performance and throughput.
Where Cray seems most intent on differentiating is providing a scalable platform that solves much larger and more interesting problems. And that means the system interconnect has to be able to handle these large training datasets across a scalable machine. “There are certainly machine learning problems that can take advantage of tens to hundreds to over a thousand GPUs,” Scott said, “and there will be a different number of customers at each of those points along the way.”
That would be something of a departure from what is currently available. Even though the training of these deep learning neural network is mostly being done at hyperscale companies, the training is not performed at hyperscale, or in most cases even at the scale of more than a handful of nodes. That has to do more with the slowness of the system interconnect than any inherent limitation to ramp up the training applications themselves.
Scott also believes his company has advantages on the software side. Cray engineers have had a lot of experiencing in tailoring communication libraries, as well as those in math and science, to work well at scale. There is also an art to structuring applications in such a way as to maximize execution across a given set of hardware. Scott says they can apply this same expertise for deep learning applications as they have for classic HPC codes in simulation and modeling.
Given all that, it’s still not clear what will Cray actually has in mind here. Will it be a souped-up CS-Storm that relies on more conventional InfiniBand or Ethernet technology to lash the GPU nodes together? Or will it be an XC series supercomputer, complete with Aries interconnect technology. The former presents a more straightforward engineering path inasmuch as that design already provides the kind of GPU density consistent with deep learning machinery.
On the other hand, building a true XC-style supercomputer with more than one or two GPUs is going to take some serious reengineering since that platform currently relies on processor daughter cards to squeeze in coprocessors. However, with Aries, the XC provides better scalability and system bandwidth, and by extension, better product differentiation compared to rival deep learning offerings.
Whatever form this platform takes, its development appears to be underway. According to Scott, they have already done some “investigation” along these lines and their initial results look “very promising.” That suggests the introduction of a deep learning offering is not too far off. With the introduction of NVIDIA’s P100 and the competitive landscape for this market starting to take shape, Cray can’t wait too long.
From his perspective though, Scott seems content not to get out too far ahead of the curve. The deep learning technology may be fast-moving, but the customers continue to operate at a human pace. “We’re really just at the beginning of the whole deep learning market,” he said.