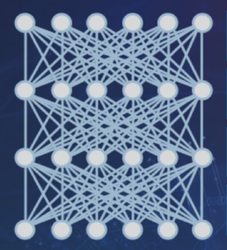
Nov. 28, 2016
By: Michael Feldman
At Intel’s recent AI Day, the chipmaker previewed a series of future products that it intends to use to unseat GPUs as the de facto standard for machine learning. The one-day event was Intel’s most assertive pronouncement of its intentions to become a major player in the artificial intelligence market.
Perhaps the most surprising element of the roadmap is the productization of the Nervana Engine, the machine learning chip that Nervana engineers were working on prior to Intel’s acquisition of the company earlier this year. Given Intel’s devotion to Xeon Phi, we at TOP500 News were more than a little skeptical that the company would ever commercialize the Nervana designs. But that is apparently the plan.
In the first half of 2017, the chipmaker intends to produce first silicon of “Lake Crest,” a deep learning accelerator that is purpose-built to train neural networks. The design is derived directly from the Nervana Engine, right down the second-generation high bandwidth memory (HBM2), which will be integrated on the package. From software memory management to eclectic math, there is nothing general-purpose about this chip.
Regarding the latter, Lake Crest will employ something called Flexpoint, a numerical format devised solely for deep learning codes. According to Naveen Rao, former Nervana CEO and now the GM of Intel's AI Solutions group, Flexpoint is neither fixed point nor floating point, but “something in between.” Rao says it allows for much higher levels of computational density and lower power per operation on deep learning training codes. As a result, he claims the Lake Crest chip will deliver up to 10 times the parallelism compared to the most advanced GPUs of today.
Lake Crest schematic. Source: Intel
Another unique aspect of Lake Crest is the absence of caches. All local memory will be managed in software, the idea being to deliver the maximum amount of data throughput from memory accesses with the least amount of expended energy. It’s a model that HPC architects have bandied about for some time, but the design never found a foothold in general-purpose processors.
The HBM2 memory capacity on Lake Crest will be 32 GB. That is twice the capacity of the latest NVIDIA P100 GPU devices. Aggregate bandwidth will be up to 8 terabytes per second, which works out to about 12 times faster than an equivalent amount of DDR4 memory. The larger capacity and the faster access are there to enable larger training models.
Inter-chip communication is supplied by 12 links of high bandwidth interconnectivity. Although no specs are currently available on the technology, it is supposed to be about 20 times the speed of PCIe and will support highly scalable configurations.
The scalability aspect is something Intel came back to repeatedly when talking about its hardware roadmap. One of the current limitations of building bigger, more complex training models is being able to scale them across large numbers of nodes. According to Intel Fellow Pradeep Dubey, they are looking to build neural networks with tens or hundreds of trillions of parameters – something out of reach on current hardware. It’s not enough to build more powerful chips, they need to be connected in high-performance fabrics to be able to scale well. like other HPC applications, machine learning has an insatiable appetite for computation, certainly beyond anything Moore’s Law can deliver.
On the inference side of machine learning, Intel will rely on a combination of Xeon CPUs and FPGAs. Initially this means Intel’s Arria 10 FPGAs, but that will undoubtedly change over time. The Xeon-FPGA combo is one that fits well into the kind of cloud datacenter where much inferencing work will go on. It offers a lot of throughput for a relatively small amount of power, something Microsoft discovered early on and is using to its advantage.
Where Intel’s AI strategy gets muddled is the Xeon Phi line. At the same time the company will be pushing Lake Crest into the market, it will also be delivering Knights Mill, the Xeon Phi variant designed for machine learning. For its part, Knights Mill is supposed to offer about four times better performance than Knights Landing on deep learning workloads, some of which is attributed to the addition of half precision floating point (FP16) arithmetic. But both Knights Mill and Lake Crest are geared to training the neural networks and both are promising superior scalability compared to their GPU competition.
Source: Intel
There are differences between the two platforms. Lake Crest will be a discrete accelerator, while Knights Mill will be a standalone bootable processor. Also, Knights Mill will retain its x86 heritage, while Lake Crest presumably will be non-x86. Although Intel didn’t say, one gets the feeling that Lake Crest will be the more powerful of the two for this training work. Perhaps that’s enough differentiation to command separate application spaces, but Intel didn’t do much at the AI Day event to clarify how they will be positioned as they hit the market next year.
Another element of that confusion is Intel’s plan to deliver “Knights Crest” somewhere in the 2020 timeframe. While the name suggests that it’s some sort of hybrid between a Xeon Phi and Lake Crest, it’s actually a Xeon CPU integrated with a Lake Crest accelerator (or, more likely, the second generation of that architecture). That gives Intel a bootable Nervana chip, which is probably what they wanted all along if they been able to secure the transistor real estate to put both on a singel die in 2017. Intel is claiming Knights Crest will deliver a 100x performance improvement on neural net training compared to the fastest GPUs today. If true, that would be a tremendous achievement, but it’s worth considering how much performance progress GPUs will also be able to make in that same timeframe.
While Lake Crest and Knights Crest will entail plenty of technical challenges, the more interesting risk for Intel is the commercial viability of such products. For any special-purpose processor, there has to be a reasonably sized market to pay for the R&D effort to create it. The machine learning market has tremendous upside, but for the time being, especially on the training side, the revenue is still relatively small. Intel must have made that calculation, probably even before it acquired Nervana, and figured it was worth the risk. The price tag for Nervana, where the original design work was performed, was about $400 million, but it will take more than that to bring the chip to market.
In any case, Intel was not about to let NVIDIA run away with AI processor market unchallenged. In the longer term, as machine learning infiltrates the analytics space and then swallows it, AI is poised to reshape much of the infrastructure in cloud and enterprise datacenters. For a company like Intel, sitting this one out was never an option.